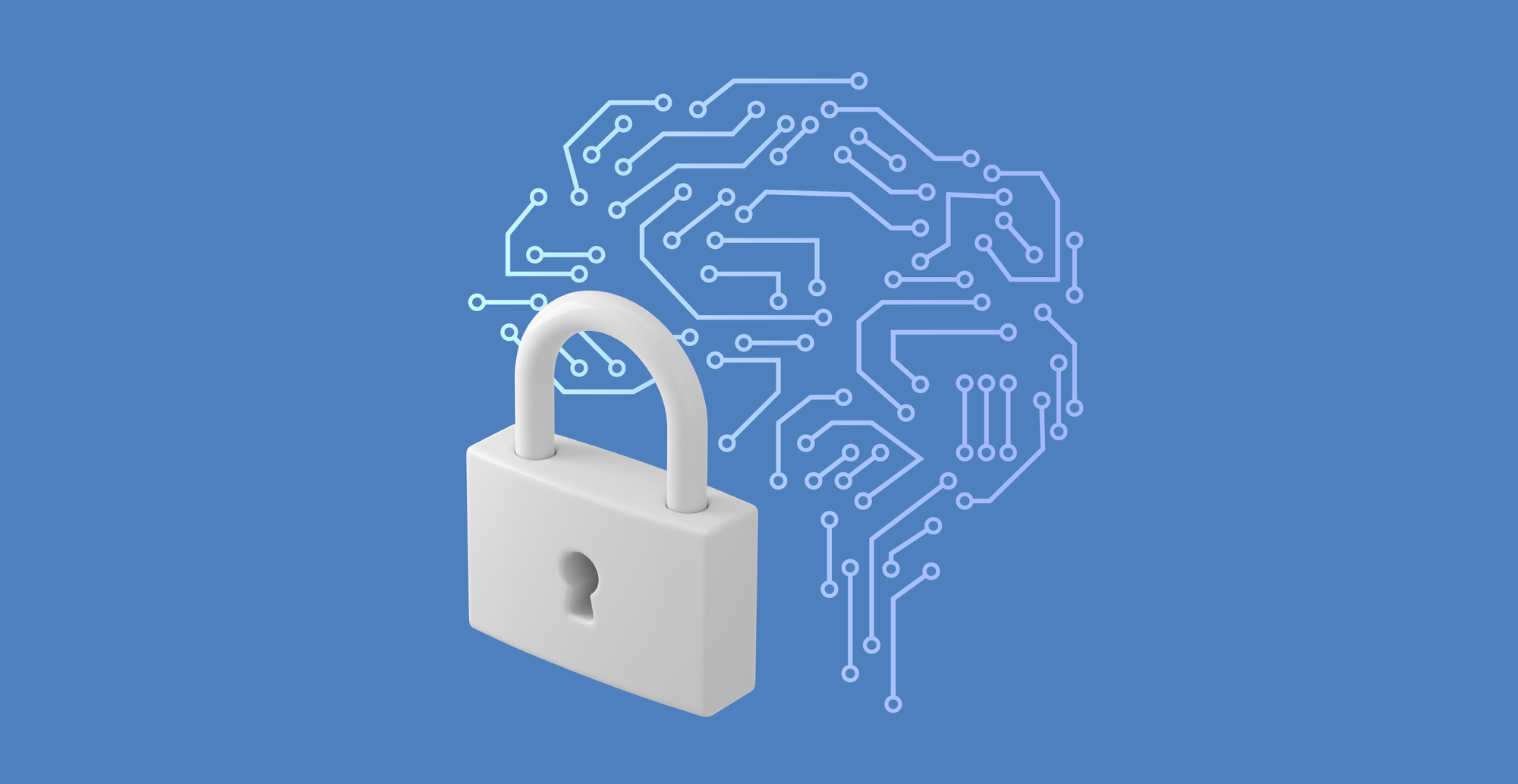
Neural networks are increasingly penetrating various spheres of our lives: from big data analysis, speech synthesis, and image creation to controlling autonomous vehicles and aircraft. In 2024, Tesla developers added neural network support for autopilot, AI has long been used in drone shows to form various figures and QR codes in the sky, and marketers and designers apply AI in their work to generate illustrations and text.
After the release of ChatGPT at the end of 2022 and its popularity, various companies have been actively developing their services based on GPT models. With the help of various services and AI-based Telegram bots, neural networks have become accessible to a wide range of users. However, if information security rules are not followed, the use of various services and neural networks involves certain risks. Let's discuss these in more detail.
Risks of using neural networks
The euphoria caused by the discovery of GPT chat for many people has been replaced by caution. With the emergence of numerous services based on language models, both free and paid, users have noticed that chatbots can provide unreliable or harmful information. Particularly dangerous is incorrect information regarding health, nutrition, and finances, as well as data on weapon manufacturing, drug distribution, and more.
Moreover, the capabilities of neural networks are constantly expanding, and the latest versions can create remarkably believable fakes, synthesizing voice or video. Fraudsters use these features to deceive their victims by forging messages and calls from acquaintances and videos with famous personalities.
The main threat is the emerging trust many users have in neural networks and various chatbots in particular. Surrounded by an aura of accuracy and impartiality, people forget that neural networks can operate on fictional facts, provide inaccurate information, and generally make erroneous conclusions. It has been shown repeatedly that mistakes happen. If you ask frivolous questions, the damage will likely be minimal. But, if you use chatbots to resolve issues in finance or medicine, the consequences can be quite destructive. Moreover, often to get a response from a neural network, you must provide some data.
A big question is how this data will then be processed and stored. No one guarantees that the information about you that you included in the queries will not subsequently appear somewhere on the darknet or become the basis for a sophisticated phishing attack.
In March 2024, bug hunters at Offensive AI Lab, thanks to a data encryption feature in ChatGPT and Microsoft Copilot, found a way to decrypt and read intercepted responses. Regardless of how quickly OpenAI was able to patch this hole, its existence is a prime example of how malicious actors might use vulnerabilities in APIs to steal confidential data, including passwords or corporate information. In addition, vulnerabilities make it possible to conduct DDoS attacks on the system and bypass protection.
There are several types of attacks on AI, and it is important to be able to distinguish them. For example, evasion attacks (modification of input data) are potentially the most frequent. If the model requires input data for operation, it can be modified appropriately to disrupt the AI. On the other hand, data poisoning attacks have a long-term character. A Trojan present in the AI model remains even after it is retrained. All this can be combined into adversarial attacks – a way to deceive a neural network to produce an incorrect result.
Neural networks are still not sufficiently protected from attacks, data falsification, and interference in their operation for malicious purposes, so users should be vigilant and follow certain rules when working with chatbots.
Precautions and recommendations
The technology of large language models is rapidly developing, penetrating deeper into everyday life, and gaining more users. To protect yourself and your data from potential threats, it is important to adhere to some rules when working with neural networks:
- Do not share confidential information with chatbots;
- Download neural network applications and services from reliable sources;
- Verify the information provided by the chatbot.
Moreover, the main recommendation when working with public neural networks is not to assume that your dialogue with it is private. It's better to avoid a situation where the questions asked contain any private information about you or your company. The exception is if you are working with an isolated instance of a neural network, located in your environment and for which your company is responsible.
Also, carefully check the services through which you interact with the neural network. An unknown Telegram channel promising free work with all known LLM models definitely should not be trusted.
Companies, whose employees use neural networks at the workplace, should be especially cautious. The interest of malicious actors in corporate data is higher, and they hunt for sensitive organizational information first and foremost.
The best way to protect against cyber threats is to implement ongoing cybersecurity and AI training for employees. This is an important component of any work process, especially in Russia, where there is a shortage of qualified cybersecurity specialists. Only 3.5% fully meet the current requirements for a worker in this field. Through training, it is possible to improve specialists' skills and, consequently, reduce the number of attacks by more than 70%.
Additional measures should also be taken to enhance the overall IT security of the company. First of all, it is necessary to develop improved AI training algorithms considering its potential vulnerabilities, which will make the model more reliable by 87%. It is also necessary to "train" the neural network: to allow it to cope with artificially created cyber attacks to improve the algorithm's performance. This will help reduce the number of hacks by 84%. Moreover, it is necessary to constantly update the software to reduce the number of vulnerabilities by more than 90%.
Conclusion
Both businesses and ordinary users have already managed to "taste" the benefits of neural networks. In a large number of areas, they help solve everyday tasks and save effort and money. For example, generative neural networks have significantly affected the cost of producing movies, TV series, and other videos where graphics and processing are needed. At the same time, roughly the same neural networks have caused a wave of deep fakes, such as the new variation of the Fake Boss attack.
Every user must understand that the neural network is vulnerable. Just like a messenger, mailbox, or work task planner – it can be hacked or subject to other failures, so it is important to approach working with it consciously.