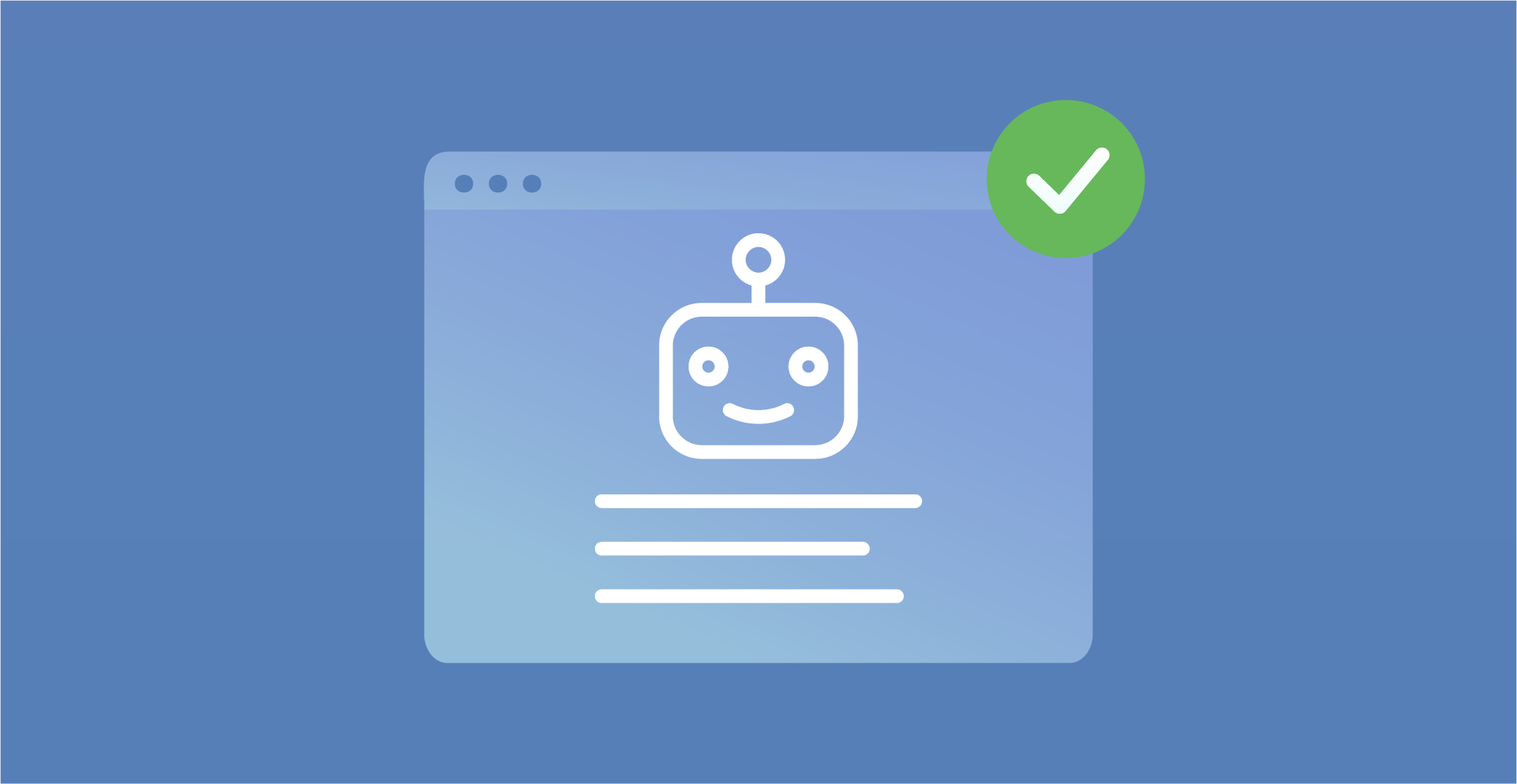
AI technologies are changing industries fast and most companies are already using or will use AI in the next few years. While AI brings many benefits — increased efficiency, customer satisfaction and revenue growth — its also introduces unique risks that need to be addressed proactively.
From reputation damage to compliance violations and cyber attacks, the consequences of poorly implemented AI systems are severe. The rise of cyber-physical systems, like autonomous vehicles, highlights the need to integrate robust safety measures into AI development and deployment.
So experts have created practical recommendations to help organizations navigate these challenges. These guidelines are designed to make sure AI systems are secure, reliable and aligned with regulatory and ethical standards so businesses can use AI safely and responsibly.
Key risks to consider
With AI being applied in so many areas, businesses need to consider many risks:
Risk of not adopting AI
This may sound counterintuitive, but assessing the gains and losses of AI adoption is key to understanding and managing other risks.
Regulatory compliance risks
Rapidly evolving AI regulations make this a dynamic risk requiring frequent reassessment. Beyond AI-specific regulations, organizations must also consider associated risks, like violations of personal data processing laws.
ESG risks
These include social and ethical concerns surrounding AI and risks of exposing sensitive information.
Risk of AI misuse
From silly to malicious use cases, users will use AI in unintended ways.
AI models and training datasets threats
Attackers will target the data used to train AI systems and compromise their integrity.
Company services integrating AI threats
These will impact the broader IT ecosystem.
Data security risks
The data processed within AI-enabled services may be vulnerable to attacks.
The last three categories encapsulate the challenges of traditional cybersecurity in complex cloud infrastructures: access control, network segmentation, vulnerability management, monitoring, supply chain security and more.
Aspects of safe AI deployment
Safe AI deployment requires a balanced approach that combines both organizational and technical measures. These can be categorized into the following areas:
Organizational measures
Employee training and leadership education. Educate staff and leadership on AI risks and mitigation tools so you have an informed workforce to manage AI challenges.
Supply chain security. Scrutinize the source of AI models and tools. Make sure all resources come from verified, secure providers to reduce vulnerabilities.
Technical measures
Infrastructure security. A robust security infrastructure is necessary, incorporating identity management, event logging, network segmentation, and advanced detection tools like Extended Detection and Response (XDR).
Testing and validation. Thorough testing ensures AI models comply with industry standards, remain resilient to improper inputs, and meet specific business requirements.
Bias detection and correction. Detecting and addressing biases, especially when models are trained on non-representative datasets, is key to fairness and accuracy.
Transparency mechanisms. User-friendly systems for reporting vulnerabilities or biases helps organizations to build trust and improve AI systems over time.
Adaptation and compliance
Timely updates and compatibility management. Structured processes for updates and compatibility are needed to keep up with the fast pace of AI evolution.
Regulatory compliance. Staying aligned with emerging AI laws and regulations is an ongoing effort, requiring dedicated resources to ensure compliance with latest standards.
Practical implications
Deploying AI means focusing on risk management and security. Identifying vulnerabilities early through threat modeling allows businesses to address potential issues before they become problems. This proactive approach reduces the likelihood of costly mistakes and ensures smoother integration of AI systems.
A secure infrastructure is equally important. By implementing strict access controls and continuous monitoring, businesses can safeguard both their AI models and the IT environments they run in. Security measures must go beyond the models themselves, protecting the entire ecosystem that supports AI functionality.
Employee training plays a big role in responsible use of AI. Teams need to work effectively with these systems and leadership need to understand the risks and manage them. Proper preparation ensures a company-wide culture of accountability and awareness.
Thorough testing and validation of AI models are non-negotiable. These processes ensure that the systems perform reliably under different conditions and align with ethical standards. Testing uncovers weaknesses in data handling and decision-making, which can be addressed before the systems go live.
Supply chain security is another key element. Organizations must carefully vet their providers and ensure all AI models and tools come from trusted sources. This reduces the risk of vulnerabilities introduced through third-party dependencies. Addressing biases in AI models, such as those stemming from unrepresentative datasets, is equally crucial for maintaining fairness and reliability.
To maintain long-term system integrity, companies should have clear processes for reporting vulnerabilities. Allowing users and external experts to report issues improves system reliability over time. Regular updates and prompt fixes for compatibility issues are essential, as the fast pace of AI development means libraries and tools change quickly.
By integrating these practices, businesses can achieve a balance between leveraging AI’s potential and managing its inherent risks.
The road ahead
AI offers immense potential, but its successful and safe deployment requires foresight, planning, and continuous vigilance. By managing risks proactively, companies can harness AI’s benefits while minimizing its risks. Moreover, collaborative efforts between policymakers, industry leaders and researchers are essential to creating a safer and more innovative AI ecosystem.